Synthetic Data for Deep Learning
Using 3D computer models to generate synthetic training data for deep learning is a rapidly expanding area of expertise. Our group has pioneered these methods for plant modeling:
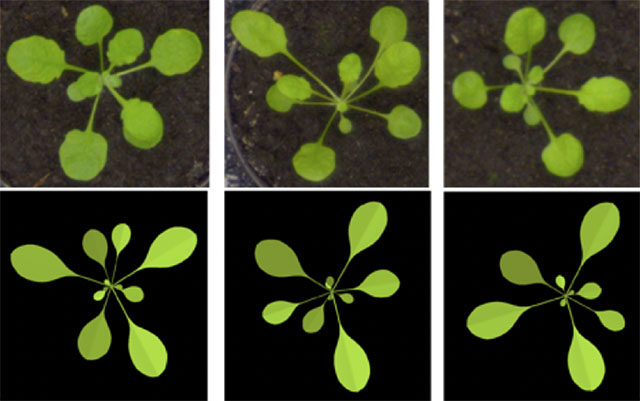
Ubbens, J., Cieslak, M., Prusinkiewicz, P., & Stavness, I. (2018). The use of plant models in deep learning: an application to leaf counting in rosette plants. Plant Methods, 14(1), 6.
ArtiSynth
ArtiSynth is a 3D biomechanical simulation platform, developed at UBC, directed toward modeling orofacial (OPAL) anatomy.
I am an active ArtiSynth developer working on advanced finite-element muscle modeling. During my PhD, I worked on three projects using ArtiSynth: a tracking-based controller for finite-element models, a model of coupled jaw-tongue-hyoid biomechanics, and a model of jaw biomechanics after surgical alteration.
OpenSim
I am an OpenSim Fellow, one of 25 world wide experts in musculoskeletal modeling & simulation. OpenSim is a musculoskeletal simulation toolkit, developed at Stanford University, directed toward modeling whole-body biomechanics.
My group develops and evaluates new musculoskeletal simulation techniques. For example, we have developed a new general formulation for muscle-wrapping over multiple smooth surfaces that provides high performance muscle paths for simulation of musculoskeletal dynamics. My group also has a number of on-going studies applying advanced muscle models to study human balance and movement with the overarching goal of trying to reduce fall-related fractures in older adults.