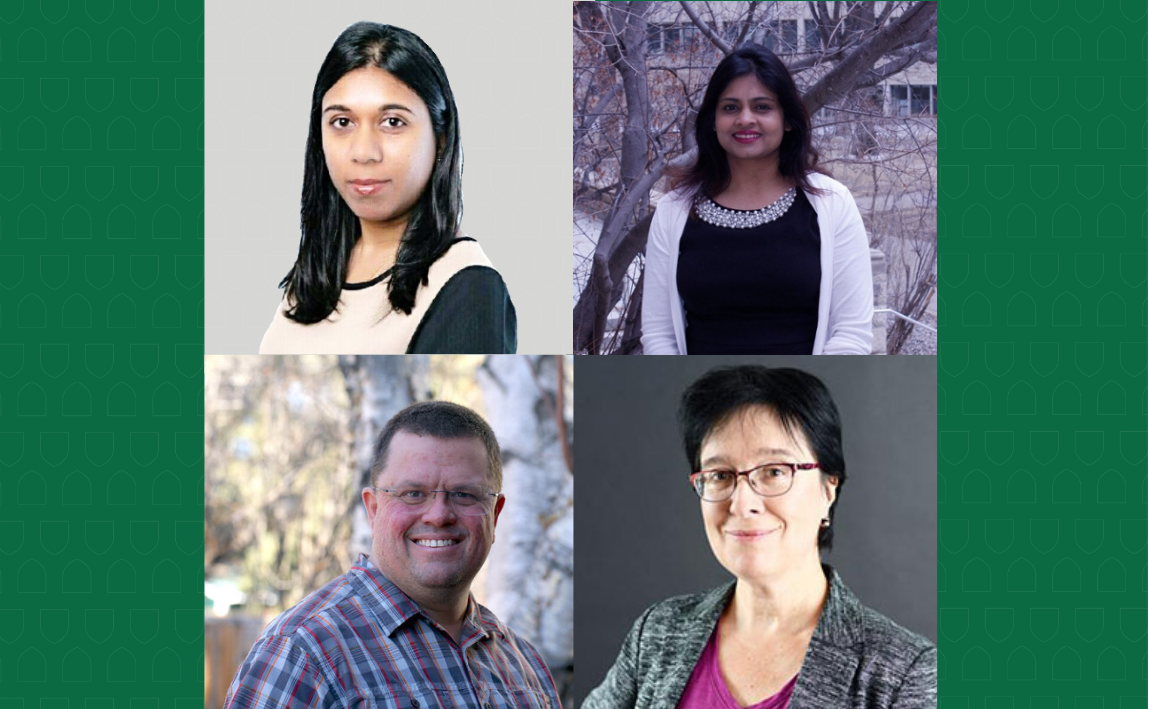
USask computer science researchers awarded NSERC Discovery Grants
Four researchers in the Department of Computer Science have been awarded NSERC Discovery Grants, totalling almost $750,000. The Discovery Grants Program provides up to five years of support to ongoing and long-term research projects which present creativity and innovation.
Dr. Zadia Codabux (PhD) - $132,500
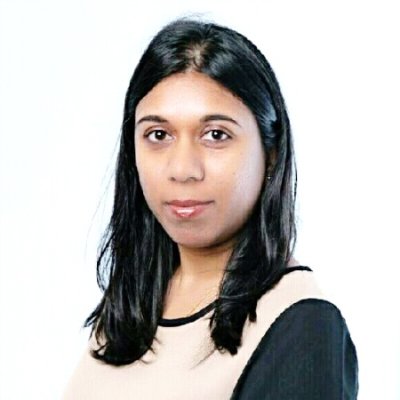
Improving software quality by managing technical debt
Technical debts are trade-offs made during software development for short-term benefits such as the expedient release of the software, but can have disastrous long-term consequences (e.g., increased defects and security vulnerabilities).
This research will contribute to more sustainable decision-making during software development to improve quality and help developers better manage resources to focus on problem areas in software systems.
Dr. Mark Eramian (PhD) - $120,000
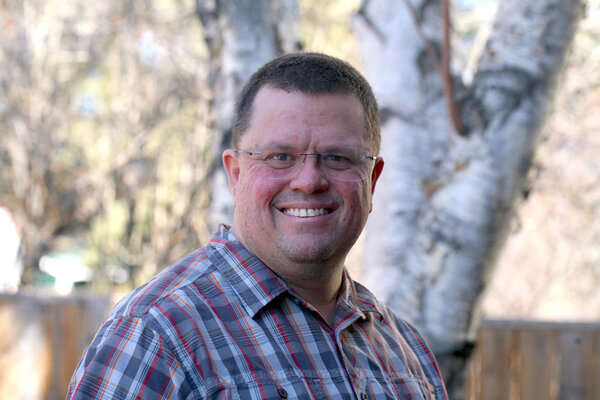
Efficient and Reproducible Image Annotation for Supervised Deep Learning with Small Data
Machine learning is currently one of the hottest and most quickly evolving technologies. Everyone wants to use "deep learning" to develop systems for everything: face recognition, identity tracking, quality control in manufacturing, tracking objects in videos, diagnosing disease, precision agriculture, etc. Deep learning works best when the machine learning algorithm can learn from a very large numbers of images where the "correct answer" is known. It is relatively easy for people to tag an image of a car, a boat, or a plane, or even different types of cars with an appropriate label. It is much more challenging to create large datasets to train systems for diagnosing cancer, or counting the number of flowers on a plant. Labeling medical images requires highly experienced experts. When marking all of the flowers on a plant, it is easy to miss one, or for a flower to be partially occluded by a leaf or another flower, or to be imprecise in specifying a flower's exact location. Moreover, in order to obtain enough annotated images to learn from, typically multiple annotators label a dataset but each image is annotated by only one annotator. In such circumstances we have no way of determining whether different annotators are annotating consistently, that is, we can't quantify the inter-annotator agreement. Annotator disagreement arises from biases in their annotations, and degrades the quality of the training dataset. Inter-rater agreement is affected by the difficulty of the annotation task and the nature of the instructions given to annotators. If we can find ways of obtaining more consistent annotations across multiple annotators, training dataset quality will improve, and hence the performance of the learned system also improves.
The proposed research program will study how to obtain better quality annotations from annotators with higher inter- and intra-annotator agreement. We will create augmented annotation tools that provide problem-specific semi-automation to assist annotators and quantify the resulting benefits to annotator agreement and trained system performance. We will quantify the relationship between annotator agreement and model performance. We will explore the degree to which contextual factors such as annotation type, instructions given, pressure, and distractions can influence annotator agreement and develop best practices for mitigating their effects.
By studying factors that influence annotator agreement and the performance of the systems that are trained from annotated datasets, we will be able to develop new standardized methodologies for training "deep learning" models with limited data. This will allow better prediction of the optimal amount of resources to invest in annotation, reduce the reliance on trial-and-error methods to obtain the best trained system performance, and make successful machine learning less reliant on deep technical expertise.
Dr. Banani Roy (PhD) - $132,500
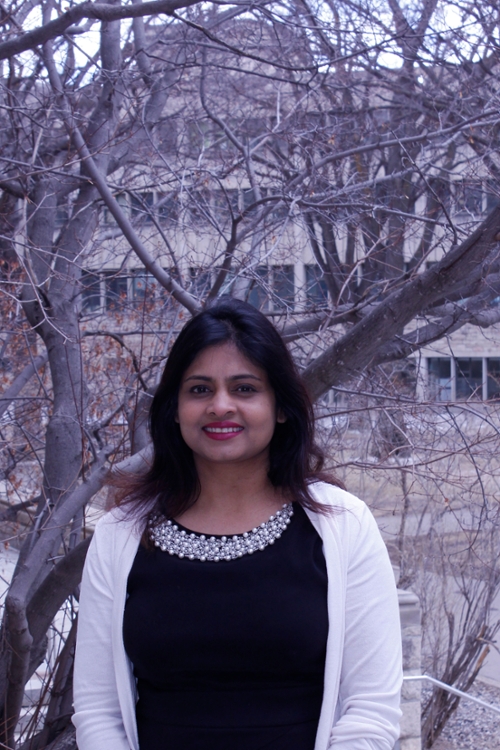
Supporting complex workflows for data-intensive discovery collaboratively, reliably and efficiently
Scientific software capable of efficiently and effectively analyzing large-scale data has become necessary for research programs exploring some of the world’s most pressing and complex challenges. As the volume, variety, and velocity of data increases, Big Data experiments making use of scientific workflow management systems (SWfMSs) to explore data, plan experimental execution, and visualize the results are becoming common and unavoidable. However, existing SWfMSs do not yet support the needs of the global scientific community to efficiently construct and adapt complex workflows, synchronously and asynchronously. As well, SWfMSs poorly handle group awareness and workflow provenance necessary for effective collaboration among distributed scientists, tracking of changes and debugging errors in workflows. The proposed research addresses these shortcomings by giving scientists methods to collaboratively and easily model their scientific experiments, understand and debug workflows, help them to recover from errors quickly, and increase their productivity. The long-term objective of this research is to develop a support framework that allows multi-disciplinary scientists to share complex workflows for data-intensive discoveries. The proposed research program will train 8 HQP: 1 PhD, 5 Masters and 2 undergraduate students. The HQP will collaborate with multidisciplinary scientists and/or industrial partners, experiencing the challenges scientists face daily and learning how they could address those challenges, as well as improving their professional skills and expanding their professional network.
The results of this research will significantly increase the productivity of scientists in several domains (including Global Climate Change and Water Security) and will pave the way for extending the ideas to other scientific domains. Furthermore, the proposed solutions will allow software engineers to leverage both the principles and methodologies of software engineering and HCI to design reusable software architecture, plugins, and graphical user interfaces, targeting the seamless interaction between scientists and complex workflows in data intensive discoveries.
Dr. Julita Vassileva (PhD) - $352,100
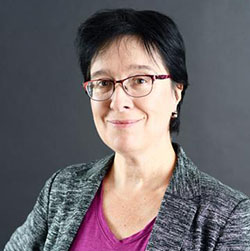
Towards Accountable and Transparent Personalized Persuasive Technology
Persuasive technologies (PT) have become ubiquitous. They are used to engage users in social networks and in apps that help people improve their health, reduce waste, save energy, manage spending, and many other beneficial uses. In our previous work we have shown that Personalization increases significantly the effectiveness of PT, and we proposed various user features and methods to design Personalized PT (PPT) in different domains. However, ethical concerns were raised in recent years culminating in the 2019 US Congress Hearing on Persuasive Technologies. The main concerns are that PPT are not transparent, can be too engaging and manipulative, and can be used for exploitative purposes. So far only legislation measures have been discussed, yet there are no technologies yet for “taming” PPT, and banning them entirely is not possible and desirable.
The long-term vision of the proposed research is to develop computational methodologies, frameworks, techniques and tools that support transparency, accountability and control of PPT. This vision will be pursued through two long-term objectives:
(Objective 1) Make PPT auditable and transparent and develop a framework supporting a market for certification and control of personalized PT.
(Objective 2) Develop methods to automatically negotiate on behalf of the user the acceptable persuasive purposes, strategies, and data used for personalization and ensure transparency and control by the user.
This research will develop methods for creating trust certificates for PPT. Furthermore, automatic methods for analysing the certificates and visualizing them to the user will be developed, ensuring transparency, as well as methods to compare them with the stated user preferences allowing the user to disable risky PPT. We will also develop methods and tools for automatic negotiation of admissible persuasive strategies according to the user's preferences, to allow strategies that are not manipulative, or are aligned with the user's goals. Finally, we will develop tools for users to inspect their personalization models, and will use these tools to stimulate users' self-awareness and help them overcome their cognitive biases.People are currently losing millions of hours of productivity, rob their loved ones from real interaction, and spend their money on things they do not need, because they are manipulated by stealth exploitative PPT. My research will create tools to make these technologies transparent and controllable by users and auditable/controllable by authorities. There has been no previous work on computational techniques making PPT accountable and transparent to the user. My research will provide training for 12 HPQ and will influence other researchers to work in this area. Thus the proposed research is ground-breaking. The resulting methods, technologies and tools will profoundly impact how people use social media and persuasive applications to improve their health, learning, efficacy and the environment.